A Diatribe against Data Contracts and their Abuses.
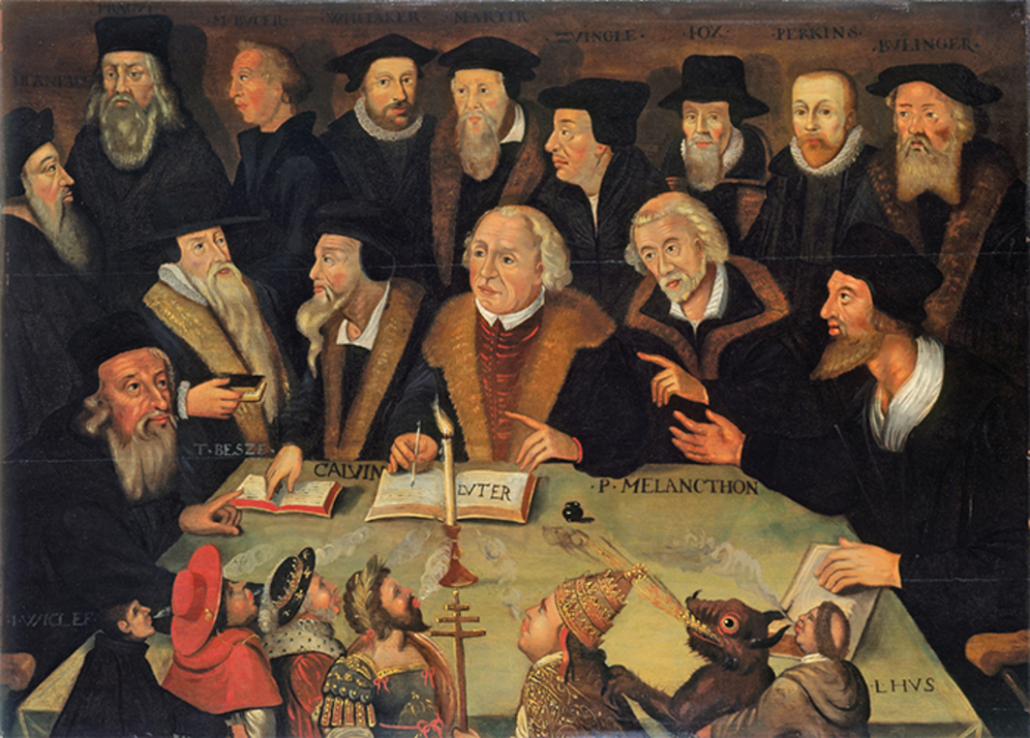
Ok, so I don’t really mean all that. Or do I? I have no idea what the future holds. Sometimes it’s easy to pick out the winners, like Databricks and Snowflake, you can see, feel, and taste the results of those data products, a delicious and delectable bounty to feast upon. Other things are harder to read the tea leaves on. Kinda like Data Mesh … is it a thing, or is it not a thing? It’s hard to decern between charlatans and marketing/sales departments hocking the next Cure All Snake Oil and real life.
What about all this recent humdrum and buzz around Data Contracts? Pushed by some popular Data Engineering faces like Ananth Packkildurai and Chad Sanderson. What is all the hype about Data Contracts, are folks just pushing another tool down our throats? Is there a real issue and problem that can be solved with Data Contracts?
Read more